Last week, I had the good fortune to attend a conference in Norway. For four days, I hung out near the shore of a beautiful lake with around 300 other scholars, caught up with friends, and heard presentations. I also gave a presentation myself: an overview of a computer model I recently built with my partner in crime, Saikou Diallo. This model simulates the way that self-regulation, the basic psychological process that guides human behavior and stabilizes emotions, is affected by involvement in a religious group. Religion, after all, is an instrument for creating and enforcing rules: from tithing and fasting to attending services, religious communities by definition expect their members to behave in certain ways. This means that normal psychological models of self-regulation aren’t enough – because most psychological models focus on individuals alone.
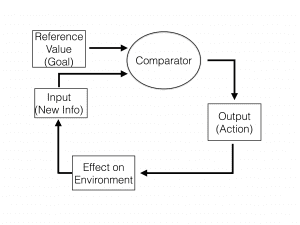
The conference was the International Association for the Psychology of Religion. The model Saikou and I created builds on probably the most well-known psychological theory of self-regulation: the cybernetic model, by Charles S. Carver and Michael F. Scheier (check out the figure at right.)
In this model, “cybernetic” doesn’t mean “has to do with computers.” It means “aimed toward a stable goal.”* A thermostat is the classic example of a cybernetic system. Say that the thermostat’s setting is, say, 70 degrees Fahrenheit. Whenever the thermostat senses that the room temperature has gone too high, it sends a signal to activate the air conditioning. If the temperature goes too low, it flips on the heat. Thanks to these constant signals and adjustments, the actual temperature of the room always stays within a comfortable range.
As a warm-blooded animal, you benefit from a similar self-regulatory process in your body, generally keeping your internal temperature within a hair or two of 98.6°.
Similarly, Carver and Scheier argue that many psychological processes and behaviors are cybernetic systems, aimed toward particular set points or goals. For instance, you might want to lose 5 pounds. You start dieting, but after two weeks, you find that you haven’t lost any weight at all. So you start exercising. A few weeks later, you find that you’ve lost 10 pounds – but that’s too much! So, again, you change your diet and exercise regime accordingly.
Other goals we strive toward might include getting up on time for work, devoting the right amount of attention to our friends and family, or learning a new language. In each case, our brains are constantly scanning for information about how we’re doing relative to our goal. If we’re not achieving it, we might start pouring more resources into our efforts. If we’re meeting it easily, we’ll slack off a bit, freeing up resources and energy to invest in other goals.
The key is that, for many goals, our self-regulation efforts are geared toward reducing the discrepancy between “ought” and “is” – between how we’re actually doing and how we’re supposed to be doing. If you want to get up each morning at 7:00 am, but you keep waking up instead at 8:00, then your self-regulation efforts will aim at reducing that one-hour discrepancy. If you move toward the goal, you’ll feel rewarded. If you move away from it, you’ll feel frustrated.
If you have a really hard time meeting your goal, then your self-regulatory process might suggest a different solution: move the goal. When you shift your objective by, say, 45 minutes, so that your new goal is to wake up at 7:45, then you’ve reduced the discrepancy between your ideal state and your actual state to only 15 minutes. It’s analogous to setting the thermostat to a higher setting during a heat wave – if you keep your house at 76° instead of 68°, the air conditioning won’t have to work as hard.
The problem is, if you can always just move your goals to make them easier to reach, then self-regulation can become self-defeating. This is why so many attempts at dieting fail: unable to reach their original goals, dieters lower their standards to a level that’s easier to achieve – but less adaptive in the long run. Same thing with exercise goals. Or efforts to quit smoking. Or resolutions to be nicer to coworkers. Self-regulation as a cybernetic process is inherently less effective or stable when the goals shift too easily.
So what happens when a goal is shared among multiple people? Well, for one thing, the goal might be more resistant to downgrading. For example, recent research has found that people who work out with partners achieve more success than people who exercise alone. In part, this is because partners encourage each other to work hard to reach their shared objectives. And nobody wants to be the weak link, so each person puts in more effort than they might otherwise.
In other words, shared goals may be more stable bases for self-regulation than individual goals.
Institutional religion, of course, is all about shared goals. If you become a member of a church or temple, the other members will apply subtle or not-so-subtle pressure on you to live up to their standards – attending services each week, volunteering, fasting, tithing. Famously, religious standards for behavior are less flexible than everyday standards. The community won’t shift its goalposts just because you find them uncomfortable. Indeed, many religious standards are sacred values, shielded from utilitarian second-guessing by an aura of spiritual significance.
So how do you build a model of something like this?
Saikou and I designed an agent-based model (ABM), in which agents (that is, simulated people) form social networks with shared goals. The agents each have their own cybernetic self-regulation processes, constantly working to help the agents reach the group goal for themselves. But there’s also a larger feedback loop between the individuals and the wider group. Namely, if too many agents fail to reach the group goal, the group goal can change.
Each agent has its own level of ability, so not every agent will be able to easily achieve the collective goal. Agents that experience social pressure to exceed their abilities become stressed, and if a group has too many stressed-out agents, it might adjust the goal to make it a little easier to achieve. One of the parameters in the model, then, is the threshold for altering the group goal: groups with higher thresholds are more resistant to change (like a religious organization).
Individual agents each also have a time horizon. This is the length of time they take into account when calculating their emotional states. Agents who are approaching the goal feel happy. Agents who are moving away from the goal feel dejected. But some agents look at their progress over, say, 10 time steps (simulated days), whereas others only look at how they’ve done over the most recent day.
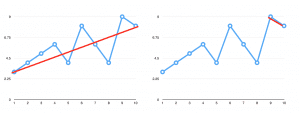
To test the model, we ran calibration experiments. In a calibration experiment, you tell the simulation engine to produce a given output, like the maximum number of stressed-out agents or the happiest agents, and then you record which combination of parameters produced that output. Our preliminary results – the heart of my presentation in Norway – were pretty interesting. When we told the computer to give us the happiest agents, it generated a combination of parameters that included a very challenging group-level goal, high average ability, and a high level of social pressure.
Interestingly, a similar combination of parameters produced the most stressed-out agents. The only difference was that average agent ability was lower. These preliminary results suggest that stress and positive emotions might not always be incompatible – in fact, social pressure to adhere to high group standards might be a key ingredient in both. (And as it turns out, moderate amounts of stress can be good for you.) But when your ability to meet high standards is low and your group puts serious pressure on you to achieve them, your well-being can take a hit.
These results might seem commonsensical, but they’re noteworthy because the simulation wasn’t designed explicitly to produce them. It was just designed to enact Carver and Scheier’s model of self-regulation in a social context. The fact that the model’s output makes good sense is a sign that Carver and Scheier’s model is onto something.
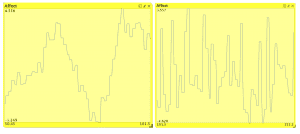
Another fascinating result was that agents with longer time horizons had much more stable emotional states. Check out the figure: the lefthand chart is an agent with a time horizon of 10 time steps, while the righthand chart is an agent with a time horizon of only two time steps. The first agent has some ups and downs. Who doesn’t? But over the course of 50 simulated days, it only experiences two peaks and two troughs. Its changes in mood are gradual.
On the other hand, the agent with a very short time horizon has many rapid ups and downs over the same length of simulated time. It also varies over a much wider range of mood levels. It’s a lot more emotionally unstable. Again, this makes intuitive sense, but it’s informative to see how a fairly simple simulation can produce this effect.
Think about someone you know who’s always going through emotional highs and lows – someone who’s not especially emotionally stable. What if that person thought more about the longer term – not how he’s doing compared to yesterday, but compared to last year or next year, or ten years down the road? He might not react to each new event as if it defined his existence.
By contrast, think of a friend or acquaintance who’s emotionally even-keeled and stable. Does she respond to each immediate disappointment or triumph as if it were the definitive statement on her life? No, probably not. Instead, she takes each new experience as just a small sliver of her overall life, reacting to it appropriately but not overreacting.
This result is important for the scientific study of religion because religion often elicits identification with much larger timescales than everyday life. My favorite example is the yearly Passover seder: it teaches observant Jews to consider their lives within a scope of time that includes events from thousands of years ago. But Christian Easter fulfills a similar function, as do the Muslim Ramadan and Hindu Janmashtami holidays. Could it be that the small but fairly consistent positive correlation between religious observance and mental health is due, in part, to the stabilizing effects of a broadened time horizon? Our simulation hints that the answer may be a qualified yes.
Of course, religion has plenty of negative effects, too. In our simulations, agents that couldn’t achieve the goals of their community were stressed out. Communities that were slower to change their standards in the face of widespread stress only exacerbated this effect. These results remind us that sometimes religious expectations are unreasonable or maladaptive, and religious communities can ask people to live up to standards that harm them. It’s hard not to think of the relationship between conservative sexual morality and religion – committed heterosexual marriage may work well for most people, but not for others. If a religious community is inflexible about its sexual norms, it may harm some people who can’t easily meet the standards of the majority. We wanted to capture this tension between strict social standards and excessive inflexibility.
This model is still in development, so our data are preliminary. But even so, they shed interesting light on what happens when goals for individual self-regulation are socially shared. They also hint at some promising avenues for studying the relationship between religiosity and mental health – especially the stabilizing effects of a longer time horizon, and the stress-inducing effects of institutional inflexibility.
_____
* The word “cybernetic” comes from the ancient Greek “κυβερνηυικός,” or “steersman” – the guy at the back of a boat who steers it.