Connor Wood
Over the past decade or so, there’s been a big groundswell in empirical research on religion. This is a good thing, because it means now we can actually point at data to answer questions about what role religion plays in culture, or whether religion is here to stay.* But just because empirical psychologists and cognitive scientists are publishing data-heavy papers on religion doesn’t mean everything they say is the gospel truth (pun intended). One recent paper shows that even our most cherished scientific conclusions can turn out to be red herrings, thanks to publication bias, cherry-picking results, and good old human error.
Self-control is a major topic of interest for scientists who study religion. High-profile studies have claimed to show that subliminal religious cues – like the word “God” flashed on a screen – can improve a person’s self-control in difficult or tedious tasks. Other studies have suggested that religion might strengthen practitioners’ self-control over time, through rituals that “exercise” our willpower.
Of course, all these claims are based on a single, hugely important premise: self-control is a finite resource. This assumption, called the “depletion model,” argues that self-control is like a muscle. Use it too much in one short period, and it gets weaker – but use it a lot over time and it gets stronger. For example, if you successfully resist the temptation of a plateful of cookies for twenty minutes straight, you might have a hard time saying “no” to clickbait links afterward. The depletion model, also known as ego depletion or the limited-resource model of self-control, is one of the most influential and celebrated theories in psychology. The original papers setting forth this idea have been cited thousands of times and replicated hundreds of times. It’s what you might call a robust effect – it persists, across studies. It’s real. You can count on it.
Or can you? Evan Carter and Michael McCullough, psychologists at the University of Miami, recently found themselves in the awkward position of being, er, unable replicate the effect. No matter how they put their experiments together, subjects weren’t showing weakened self-control after conducting willpower-draining tasks. This shouldn’t have been so hard, considering how many hundreds of psychologists have vouched for it. But it was.
WARNING – Various Statistics Terms Ahead
To figure out why, Carter and McCullough examined a meta-analysis (a quantitative review of many different studies). The meta-analysis in question, published in 2010 by another group of researchers, covered 198 different papers, and found that the self-control depletion effect was robust across studies and had a medium-ish effect size.
But there were some problems. The 198 papers showed signs of being heavily biased toward significant results. This publication bias is also called the “file drawer effect,” because negative results – which are famously difficult to get published – often languish in researchers’ file drawers. Statistically speaking, there should be some null results for every line of inquiry – even when dealing with big effects like the health impacts of smoking. Ergo: when journal databases turn up nothing but positive results for a given topic, there’s probably a file-drawer effect.
To investigate this possibility, Carter and McCullough estimated the average statistical power in the 198 studies. Using their estimate, they derived the probability that any given study would turn up positive findings. The logic is simple: strong effects are more likely to appear in any given study. If you were studying whether caffeine makes toddlers hyperactive, any randomly chosen study would be very likely to show a significant effect. But the effects of, say, decaf coffee on grownups would be more likely to remain invisible in any individual study, because the influence of wee amounts of caffeine is very subtle – even though it’s real.
Self-control depletion – according to the literature, anyway – lies somewhere between coffee and decaf. It should show up fairly regularly, but not in every study. As it turns out, 151 of the 198 studies in the meta-analysis showed significant positive effects. Given the average statistical power of those findings, Carter and McCullough calculated that the chances of so many studies achieving significance were vanishingly small, yielding an “incredibility index” of .999.† In other words, there was a 99.9% probability that there were more significant results than should be expected given the average effect size. That’s a lot of negative results stuffed in file drawers.
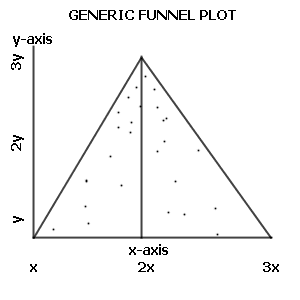
But this method of estimating publication bias can be cumbersome. To improve their guesses, Carter and McCullough conducted another test. They assumed that the precision of measurements should be inversely related to effect sizes. In other words, if your measurements are imprecise, you’re likely to overestimate how big the effect is. If you plot these two variables on a graph, they form a funnel, with overestimates of effect size increasing as your measures (standard error) get less precise.
Here’s the key: this funnel will be asymmetrical if there’s publication bias in the literature. Publications with null or negative findings will be missing, and so will studies with precise measurements. So if you want to estimate actual effect sizes, you need to extrapolate the values of the missing data points and add them to the plot.
This technique is called the “trim-and-fill method,” since you “trim” and “fill in” the funnel – that is, you add assumed results from hypothetical studies that have not been published – to make the graph symmetrical. Then you re-estimate effect sizes from there.
In addition to this basic technique, Carter and McCullough also conducted a modified version of the trim-and-fill method that uses statistical regression to determine whether the real-world effect size is probably zero. If it turns out not to be likely to be zero, a second regression analysis estimates the actual effect size.
In a recent meta-analysis of FDA pre-registered antidepressant studies, researchers used this same two-step method to accurately estimate effect sizes despite a huge file-drawer effect: 94 percent of the published papers showed significant positive findings, but only 51 percent of the total group of studies (including unpublished trials) attained significance. The published studies yielded a respectable average effect size of around .41. But using the full sample, the researchers calculated a much smaller genuine effect size of .31. Then, using the two-step trim-and-fill method, the researchers re-calculated the average effect size using only the published studies. In other words, they estimated the effects of the “missing” studies – and turned up an effect size of .29. This is, obviously, very close to .31. The upshot? The two-step trim-and-fill method – which only simulates the unpublished studies – can produce highly accurate estimates of actual effect sizes.
So what happened when Carter and McCullough applied this method to the research on self-control depletion? They estimated an actual effect size of zero. That’s right – nada. The missing studies – those null results that never got published – left a publication record so biased that the likely real-world effect was apparently negligible. But if you just scanned the psychology journals, you’d never know it.
This is an interesting case, because the self-control depletion model makes so much sense. Even Carter and McCullough admit that their own understanding of human nature leads them to believe in self-control depletion. I mean, it’s obvious: if you focus on resisting a temptation for long enough, you’ll drain your willpower and have less self-control afterwards. Right? But the evidence – if you include the data that’s rotting away in file drawers – might not support this commonsense belief.
So does Carter and McCullough’s analysis mean that self-control depletion is actually imaginary? Not necessarily. Because it matches people’s experience and fits so well with other psychological theories, researchers will be hesitant to throw it away – and that’s a good thing. If scientists tossed theories too easily, science would implode. So instead of discarding the self-control depletion model, Carter and McCullough argue forcefully for much larger studies, with more rigorous controls, to discover once and for all whether there’s actually an effect.
Since self-control is a major focus for religion researchers – Carter and McCullough themselves have published on it – their paper is more than a little unsettling for the scientific study of religion. If their results hold, many theories of religion’s influence on self-regulation may need to be seriously revised. Some may need to be scrapped altogether.
Carter and McCullough’s disquieting paper is a stark reminder that the file-drawer effect and publication bias are massive problems, especially in psychology. If researchers only publish when they get positive effects, leaving their null findings moldering in folders, then we have no idea whether the findings that splash across newspapers or find their way into textbooks are actually, you know, valid. To fix this problem, researchers should commit to publishing their null results, pre-registering experiments, and refraining from p-hacking. These new standards might require a little self-control. But if we really want to learn about these odd animals called humans, they’ll be worth it.
____
* Answer: Yep
† Yes, this is really a thing. People abbreviate it as “IC-Index.” I’d never heard of it either. You’re welcome.
____
Edit: After publishing this post, I added an example of self-control depletion and some additional terms. I woke up this morning desperately convinced that I needed to do this. Maybe one of these days I could use a vacation.